Overview of AI in Retail
Definition and Importance of AI in the Retail Sector
Artificial Intelligence (AI) in retail refers to the use of advanced computational techniques and algorithms to analyze data, automate processes, and enhance decision-making within the retail sector. This technology enables retailers to optimize their operations, improve customer experiences, and drive sales by leveraging vast amounts of data generated from various sources, including customer interactions, sales transactions, and supply chain activities.
AI has become increasingly important in the retail sector as it empowers businesses to respond swiftly to changing consumer preferences and market dynamics. With the retail landscape becoming more competitive, companies are turning to AI to gain insights from big data, allowing them to personalize offerings and streamline operations effectively. By implementing AI solutions, retailers can enhance customer engagement, improve operational efficiency, and ultimately drive revenue growth.
The integration of AI into retail processes can be seen as a game-changer, as it facilitates a more data-driven approach to strategy formulation and execution. Businesses that harness the power of AI can not only address current challenges more effectively but also anticipate future trends, thereby positioning themselves at the forefront of innovation in the retail space. As a result, AI is not merely a technological enhancement; it represents a fundamental shift in how retailers operate and interact with their customers, making it an essential component for success in today’s digital economy.
Current Trends in AI Adoption among Retailers
The landscape of retail is rapidly evolving, with artificial intelligence (AI) at the forefront of this transformation. Retailers are increasingly adopting AI technologies to enhance operational efficiency, improve customer engagement, and drive sales growth. One of the most significant trends in AI adoption is the use of machine learning algorithms to analyze consumer data and predict shopping behaviors. This analysis allows retailers to tailor their offerings and marketing strategies to meet the specific needs and preferences of their customers more effectively.
Another noteworthy trend is the integration of AI into customer service operations. Retailers are leveraging chatbots and virtual assistants to provide immediate support to shoppers, enhancing the overall customer experience. These AI-driven tools can handle inquiries, recommend products, and assist with purchases around the clock, allowing retailers to engage with customers in a more personalized and timely manner.
Moreover, the utilization of AI for inventory management is gaining traction. Retailers are employing predictive analytics to forecast demand more accurately, reducing the risks associated with overstock and stockouts. This data-driven approach not only optimizes inventory levels but also improves cash flow and reduces waste, thereby contributing to a more sustainable retail operation.
Dynamic pricing models are also emerging as a critical trend, with retailers using AI to adjust prices in real-time based on market conditions, competitor pricing, and consumer demand. This flexibility in pricing strategies enables retailers to maximize profit margins while remaining competitive in a fast-paced marketplace.
Furthermore, the rise of omnichannel retailing is influencing AI adoption. Retailers are increasingly implementing AI solutions that provide a seamless shopping experience across various channels, including brick-and-mortar stores, e-commerce platforms, and mobile applications. This holistic approach allows retailers to gather insights from multiple touchpoints, further refining their strategies to enhance customer satisfaction.
In summary, the current trends in AI adoption among retailers highlight a shift towards more intelligent, data-driven operations. As technology continues to evolve, retailers are poised to leverage these advancements to foster innovation, enhance customer experiences, and ultimately drive growth in a competitive industry.
Enhancing Customer Experience

Personalized Shopping Experiences
Recommendation Engines
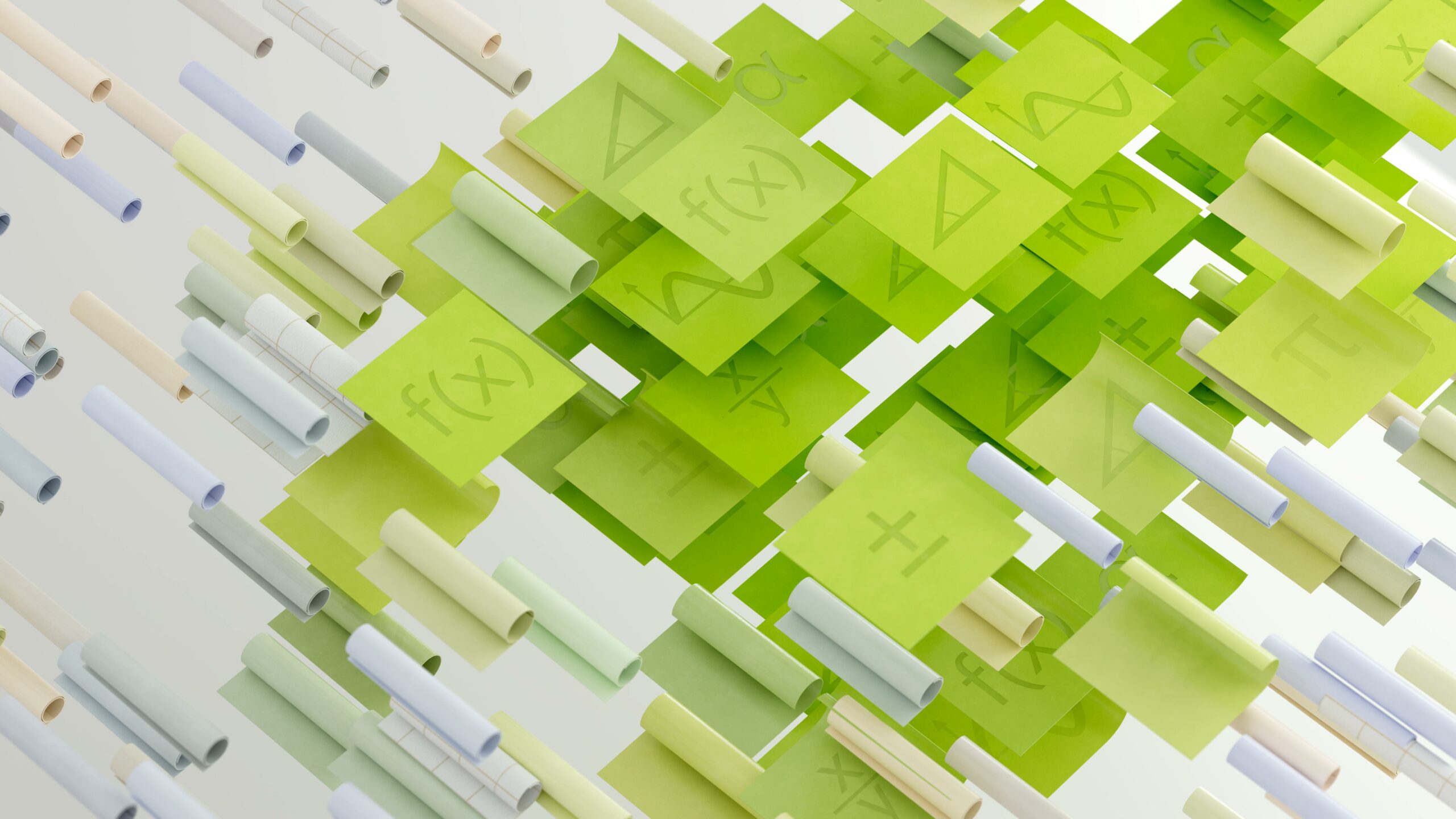
In the rapidly evolving landscape of retail, recommendation engines have emerged as a pivotal technology that enhances the shopping experience for customers. These sophisticated algorithms analyze vast amounts of data to provide personalized product suggestions, thereby making the shopping journey more engaging and relevant. By leveraging customer behavior, purchase history, and even browsing patterns, retailers can create tailored recommendations that resonate with individual preferences.
For instance, when a customer logs onto an e-commerce platform, the recommendation engine processes data points such as past purchases, items frequently browsed, and even similar customer profiles to suggest products that the customer is likely to be interested in. This level of personalization not only increases the likelihood of a purchase but also fosters customer loyalty, as shoppers feel understood and valued. As a result, retailers benefit from increased conversion rates and improved customer satisfaction.
Moreover, the effectiveness of recommendation engines extends beyond online shopping experiences. In physical retail environments, smart in-store technologies can utilize customer data to inform staff about which products to promote based on real-time analytics. This integration of online and offline data creates a seamless shopping experience, ensuring customers encounter relevant product suggestions regardless of the medium they choose.
Beyond simply suggesting products, advanced recommendation engines can also incorporate social proof elements, such as user reviews and ratings, to further guide customer decisions. By highlighting products that are not only popular but also have positive feedback from other consumers, retailers can instill a sense of confidence in buyers, further driving sales.
To optimize the performance of recommendation engines, continuous learning and adaptation are crucial. Machine learning algorithms can refine their predictions based on new data, ensuring that recommendations stay fresh and aligned with changing consumer behavior. As consumers engage with different products and trends evolve, recommendation engines remain agile, adapting to these shifts to maintain relevance.
In summary, recommendation engines play a critical role in creating personalized shopping experiences that cater to the unique preferences of each customer. By harnessing the power of data analytics and machine learning, retailers can enhance customer satisfaction, encourage repeat visits, and ultimately drive revenue growth.
Tailored Marketing Campaigns
In the realm of retail, tailored marketing campaigns are becoming increasingly essential as businesses strive to create meaningful connections with their customers. The advent of AI technologies has transformed traditional marketing strategies, enabling retailers to harness consumer data effectively and craft personalized content that resonates with individual preferences and behaviors.
AI-driven insights allow retailers to segment their customer base more accurately than ever before. By analyzing purchasing history, browsing patterns, and demographic information, AI algorithms can identify distinct customer segments and personalize marketing messages for each group. For example, a retailer might use AI to determine that a specific segment of their customers frequently purchases athletic wear. This insight can inform a targeted campaign showcasing the latest sports apparel, complete with tailored promotions that appeal to this audience.
Moreover, AI can facilitate dynamic content creation, adapting marketing materials in real-time based on customer interactions. For instance, if a customer regularly engages with emails that feature discounts, the retailer can prioritize special offers in subsequent communications. This level of personalization not only increases engagement rates but also enhances the likelihood of conversion, as customers are more inclined to respond to content that feels relevant to their interests.
Additionally, retailers can employ AI to optimize their marketing channels. By analyzing data from various platforms—such as social media, email, and websites—AI tools can determine which channels yield the highest engagement for specific customer segments. This leads to more efficient allocation of marketing resources and ensures that campaigns are delivered through the most effective mediums.
The integration of AI into tailored marketing campaigns also extends to predictive analytics. Retailers can utilize AI to forecast future buying behaviors based on past interactions. This predictive capability allows them to anticipate customer needs and create proactive marketing strategies, such as sending reminders for seasonal products or re-engagement campaigns for customers who have not made a purchase in a while.
In conclusion, tailored marketing campaigns powered by AI are revolutionizing the way retailers connect with their customers. By leveraging advanced analytics and personalization techniques, businesses can deliver targeted messages that not only capture attention but also drive customer loyalty and increase sales. As retailers continue to adopt and refine these technologies, the potential for creating deeper, more meaningful customer relationships will only grow.
Virtual Assistants and Chatbots
Customer Service Automation
In the retail sector, virtual assistants and chatbots are transforming customer service by automating interactions and providing instant support. These AI-driven tools enable retailers to manage customer inquiries efficiently, ensuring that shoppers receive timely responses to their questions or issues. By utilizing natural language processing (NLP), virtual assistants can understand and interpret customer queries, allowing them to provide relevant information and solutions without the need for human intervention.
One of the key advantages of customer service automation through chatbots is the ability to handle a high volume of inquiries simultaneously. During peak shopping times or promotional events, traditional customer service channels may become overwhelmed, leading to longer wait times and frustrated customers. Chatbots, however, can engage multiple users at once, delivering consistent and accurate responses, which enhances overall customer satisfaction.
Moreover, chatbots can be programmed to provide personalized experiences by leveraging customer data and purchase history. When a customer initiates a chat, the virtual assistant can access relevant information to tailor responses and recommendations, creating a more engaging interaction. For example, if a customer frequently purchases running shoes, the chatbot might highlight new arrivals in that category or offer discounts on related products, effectively upselling while addressing the customer’s specific preferences.
The implementation of AI in customer service also allows for continuous learning and improvement. As chatbots interact with more customers, they gather insights and data that help refine their algorithms, enabling them to respond more accurately over time. This process can lead to a reduction in the number of repetitive questions directed to human agents and allows staff to focus on more complex inquiries that require human empathy and decision-making.
Ultimately, the integration of virtual assistants and chatbots into retail customer service not only enhances the customer experience by providing quick, personalized, and round-the-clock support but also drives operational efficiency for retailers. By automating routine tasks, businesses can allocate resources effectively, ensuring that both customers and employees benefit from improved service levels.
24/7 Support and Engagement
The integration of virtual assistants and chatbots in the retail sector has revolutionized customer support by delivering 24/7 availability and engagement. Retailers are leveraging these AI-driven tools to ensure that customers receive immediate assistance at any time, eliminating the constraints of traditional business hours. This around-the-clock support is critical in today’s fast-paced shopping environment, where consumers expect answers and assistance at their convenience.
Virtual assistants are capable of handling a wide range of customer inquiries, from providing product information to processing returns and refunds. By utilizing natural language processing (NLP), these AI systems can understand and respond to customer queries in a conversational manner, making interactions feel more personal and engaging. The ability to understand context and sentiment allows them to provide more accurate and relevant responses, enhancing the overall customer experience.
Moreover, chatbots can efficiently manage multiple queries simultaneously, significantly reducing wait times for customers. This capability not only improves customer satisfaction but also frees up human agents to focus on more complex issues that require personal attention. By handling routine inquiries and transactions, chatbots help streamline operations and optimize resource allocation within customer service teams.
The deployment of AI-powered virtual assistants also supports proactive engagement with customers. For instance, they can initiate conversations based on user behavior, such as sending reminders for abandoned carts or suggesting complementary products based on previous purchases. This proactive approach fosters a deeper connection with customers and encourages them to engage with the brand further.
In addition to improving customer service efficiency, chatbots offer invaluable insights into customer preferences and behaviors through data collection and analysis. Retailers can utilize this information to refine their offerings, tailor marketing strategies, and enhance customer loyalty programs. The continuous learning capabilities of AI ensure that these systems become more effective over time, adapting to changing customer needs and industry trends.
Overall, the implementation of virtual assistants and chatbots in retail not only meets the demand for instant support but also enhances customer engagement and loyalty. As technology evolves, these AI solutions will likely become even more sophisticated, offering personalized and immersive shopping experiences that further redefine how retailers interact with their customers.
Inventory Management and Optimization
Predictive Analytics for Demand Forecasting
Reducing Overstock and Stockouts
Predictive analytics plays a crucial role in demand forecasting, enabling retailers to align their inventory levels with customer demand more effectively. By leveraging historical sales data, seasonal trends, and external factors such as economic indicators or weather patterns, retailers can anticipate which products will be in demand and when. This foresight allows businesses to reduce instances of overstock and stockouts, which can significantly impact their profitability and customer satisfaction.
Overstock occurs when retailers overestimate demand and order more inventory than needed, leading to surplus stock that ties up capital and incurs additional holding costs. Predictive analytics helps mitigate this issue by analyzing past purchasing behavior and market trends to refine inventory orders. For instance, if a retailer notices a consistent uptick in sales of a particular product during certain times of the year, they can adjust their inventory accordingly, ensuring they have enough stock to meet customer needs without exceeding what is necessary.
Conversely, stockouts can lead to lost sales and diminished customer trust, as shoppers are often unwilling to wait for items to be restocked. Predictive analytics enables retailers to identify potential shortages before they occur, allowing for timely replenishment orders. By analyzing patterns such as lead times from suppliers and sales velocity, retailers can place orders in advance to prevent disruption in service.
Moreover, machine learning models can enhance predictive analytics by continually learning from new data, increasing accuracy over time. These algorithms can adapt to changes in consumer behavior, emerging trends, and even competitive actions, ensuring that retailers remain agile in their inventory management. This data-driven approach not only optimizes inventory levels but also enhances overall operational efficiency, enabling retailers to respond proactively to market demands.
In summary, the integration of predictive analytics within inventory management processes significantly reduces the risks associated with overstock and stockouts. By harnessing data insights, retailers can achieve a more balanced inventory system, ultimately leading to improved customer satisfaction and increased profitability.
Data-Driven Decision Making
Data-driven decision-making in inventory management is crucial for retailers aiming to optimize their stock levels and improve overall operational efficiency. By leveraging predictive analytics, retailers can analyze historical sales data, market trends, and external factors such as seasonality and economic indicators to accurately forecast demand for their products. This analytical approach allows businesses to anticipate fluctuations in customer demand, enabling them to adjust inventory levels proactively rather than reactively.
For instance, retailers can utilize algorithms that incorporate various data sources—including past sales, local events, and even weather patterns—to identify patterns and trends that affect consumer purchasing behavior. By understanding these patterns, retailers can make informed decisions about when to replenish stock, which products to promote, and how much inventory to hold at any given time.
Moreover, data-driven decision-making goes beyond merely responding to past trends. Through real-time data analysis, retailers can implement agile inventory strategies that adapt to changing market conditions. This adaptability is particularly important in today’s fast-paced retail environment, where consumer preferences can shift rapidly. Retailers who harness the power of predictive analytics can minimize the risks of overstocking and stockouts, thus reducing holding costs and improving cash flow.
Additionally, investing in advanced analytics tools can facilitate cross-departmental collaboration. For instance, insights generated from demand forecasting can be shared with marketing, helping them align promotional strategies with anticipated inventory levels. Similarly, the sales team can use these insights to plan their outreach effectively, ensuring that they are promoting products that are in adequate supply and ready for sale.
In summary, effective data-driven decision-making through predictive analytics empowers retailers to optimize their inventory management processes. By accurately forecasting demand and aligning their inventory strategies with real-time market insights, retailers can enhance their operational efficiency, reduce waste, and ultimately improve customer satisfaction.
Automated Inventory Management Systems
Real-time Inventory Tracking
Automated inventory management systems represent a significant advancement in the retail sector, allowing businesses to achieve real-time inventory tracking with unprecedented accuracy and efficiency. These systems utilize sophisticated technologies such as RFID (Radio-Frequency Identification), IoT sensors, and cloud-based platforms to provide continuous updates on inventory levels, enabling retailers to have a clear and immediate view of their stock.
Real-time inventory tracking empowers retailers to respond swiftly to changes in consumer demand. For instance, retailers can monitor sales patterns and stock levels at multiple locations simultaneously, ensuring that popular items are replenished promptly while minimizing excess inventory. This capability not only reduces the risk of stockouts, which can lead to lost sales, but also helps to mitigate the costs associated with overstocking, such as increased holding costs and potential markdowns on unsold merchandise.
Furthermore, real-time tracking allows for enhanced visibility throughout the supply chain. Retailers can trace the movement of products from suppliers to the sales floor, providing insights into delays or disruptions that could impact inventory availability. This information facilitates proactive decision-making and allows for adjustments in purchasing or logistics strategies to better align with actual demand.
The integration of real-time inventory tracking systems also aids in improving operational efficiency. Retail staff can spend less time manually counting and checking inventory levels, allowing them to focus on more value-added activities such as customer service or merchandising. Additionally, with automated alerts for low stock levels, retailers can ensure that they replenish items just in time, which enhances the customer experience by maintaining product availability.
In summary, automated inventory management systems with real-time tracking capabilities revolutionize how retailers manage their stock. They not only streamline operations but also enhance responsiveness to market demands, ultimately leading to improved sales performance and customer satisfaction. As technology continues to evolve, retailers who embrace these systems will likely gain a competitive edge in an increasingly dynamic marketplace.
Supply Chain Optimization
Automated inventory management systems are revolutionizing supply chain optimization by providing retailers with the tools necessary to enhance efficiency, reduce costs, and improve service levels. These systems utilize advanced algorithms and real-time data analytics to streamline the various processes involved in inventory management. By integrating AI technologies, retailers can gain deeper insights into their supply chains, enabling them to make informed decisions and respond swiftly to changing market conditions.
One of the key benefits of automated inventory management is the ability to track inventory levels in real-time across multiple locations. This visibility allows retailers to accurately monitor stock levels, understand their inventory turnover rates, and identify which products are underperforming. With this data at their fingertips, retailers can adjust reorder points, avoid excess stock, and minimize the risk of stockouts, thereby optimizing their inventory levels and ensuring they meet customer demand without incurring unnecessary costs.
Furthermore, AI-driven systems can analyze historical sales data and market trends to predict demand fluctuations more accurately. By employing predictive analytics, retailers can anticipate shifts in consumer behavior, seasonal variations, or even disruptions in the supply chain, such as natural disasters or geopolitical events. This foresight empowers businesses to plan their inventory accordingly, optimizing procurement strategies and reducing waste.
Supply chain optimization through automated inventory management also extends to vendor management. AI systems can evaluate supplier performance based on various metrics, such as delivery times, quality, and pricing. By assessing this data, retailers can identify the most reliable suppliers, negotiate better terms, and maintain optimal stock levels without over-relying on a single vendor. This diversification minimizes risks associated with supply chain disruptions and enhances overall resilience.
Additionally, integration of AI with IoT devices can further enhance supply chain efficiency. Smart shelves equipped with sensors can provide real-time data on product availability and even alert staff when restocking is necessary. This level of automation not only saves time but also improves accuracy in inventory reporting, reducing human error.
Overall, automated inventory management systems powered by AI are transforming the way retailers approach supply chain optimization. By leveraging real-time data analytics, predictive insights, and efficient vendor management, retailers can enhance operational efficiency, respond promptly to market changes, and ultimately deliver a better experience for their customers. The future of retail will increasingly hinge on the ability to utilize these advancements to create a seamless and responsive supply chain.
Pricing Strategies
Dynamic Pricing Models
Leveraging AI for Real-Time Pricing Adjustments
Dynamic pricing models have become increasingly vital in the retail sector, allowing retailers to adjust prices in real-time based on a variety of external factors. By leveraging AI technologies, retailers can analyze a plethora of data points—such as customer demand, competitor pricing, seasonality, and inventory levels—to make informed pricing decisions that maximize revenue and maintain competitiveness.
AI algorithms can process vast amounts of data almost instantaneously, enabling retailers to respond to market changes quickly. For instance, if a competing store reduces its prices, AI systems can notify retailers to adjust their prices accordingly, ensuring they remain attractive to consumers. Similarly, if there is a surge in demand for a particular product—driven by factors such as a viral social media trend—AI can help increase prices to reflect the heightened interest, optimizing profit margins without alienating customers.
Moreover, these AI-driven dynamic pricing models are not limited to simple price adjustments. They can incorporate predictive analytics to forecast future demand and price elasticity, allowing retailers to set prices that align with long-term business strategies. This predictive capability is particularly useful during peak shopping seasons or special events, where demand can fluctuate significantly.
The benefits of implementing AI for real-time pricing adjustments extend beyond immediate sales. By continuously monitoring market trends and consumer behavior, retailers can build more resilient pricing strategies that adapt to changing economic conditions, ultimately contributing to sustained profitability and customer satisfaction.
Competitor Price Monitoring
In today’s competitive retail environment, dynamic pricing models have emerged as a crucial strategy for maximizing profitability and maintaining a competitive edge. A significant component of this strategy involves competitor price monitoring, which allows retailers to intelligently adjust their prices in real-time based on market conditions and competitor actions.
Competitor price monitoring leverages AI and machine learning algorithms to analyze the pricing strategies of rivals. By continuously scraping data from various sources, such as competitor websites, marketplaces, and promotional emails, AI systems can provide insights into current price points, discounts, and promotional activities. This information empowers retailers to identify pricing trends and adapt their own strategies accordingly.
For example, if a competitor reduces the price of a popular product, AI-driven systems can alert retailers, enabling them to quickly assess whether a price adjustment is necessary to remain attractive to consumers. This real-time responsiveness is essential in preventing lost sales opportunities and ensuring that customers perceive the retailer as offering competitive pricing.
Moreover, competitor price monitoring aids in the identification of pricing patterns and consumer responses. By analyzing historical pricing data and sales figures, retailers can better understand how price changes affect customer purchasing behavior. This analysis can lead to informed decisions about the optimal price points that not only encourage sales but also enhance profitability.
However, implementing effective competitor price monitoring systems comes with challenges. Retailers must ensure that the data they collect is accurate and relevant, as well as compliant with legal regulations concerning data usage and competitive intelligence. Additionally, reliance on competitor pricing must be balanced with the retailer’s brand positioning and value proposition, as constant price adjustments can impact consumer perceptions and brand loyalty.
In summary, competitor price monitoring plays a critical role in the development of dynamic pricing models in retail. By utilizing advanced AI technologies to track and analyze competitor pricing, retailers can make data-driven pricing decisions that enhance competitiveness, optimize sales, and drive profitability. As the retail landscape continues to evolve, those who harness the power of competitor price monitoring will be well-positioned to navigate the complexities of pricing strategy and achieve sustained success.
Price Optimization Techniques
Analyzing Consumer Behavior and Trends
Price optimization techniques in retail leverage AI to analyze consumer behavior and trends effectively. By harnessing vast amounts of data from various sources—such as historical sales data, customer demographics, and market trends—AI algorithms can identify patterns and preferences to inform pricing decisions.
One of the key elements in analyzing consumer behavior is understanding how different price points affect purchasing decisions. Retailers can utilize machine learning models to simulate various pricing scenarios, predicting customer response based on past behavior. For example, if data indicates that a particular demographic tends to purchase more during promotional periods, retailers can strategically time discounts or bundle offers to maximize sales among that group.
Additionally, AI tools can assess the impact of external factors—such as seasonal trends, economic indicators, and competitor pricing—on consumer purchasing habits. By integrating real-time data, retailers can adjust their pricing strategies dynamically. For instance, if a competitor lowers their prices, AI systems can analyze how that move has historically affected consumer behavior and suggest an optimal response, whether it be adjusting prices or enhancing value propositions through marketing strategies.
Understanding consumer behavior also involves segmenting customers into various categories based on their purchasing habits. AI can identify clusters of consumers with similar behaviors, allowing retailers to tailor their pricing strategies to specific segments. This segmentation enables targeted pricing that aligns with the perceived value for each group, resulting in improved sales conversions.
Furthermore, advanced analytics can help retailers forecast future trends in consumer behavior. By utilizing predictive analytics, businesses can anticipate shifts in demand and adjust prices accordingly. For example, if data suggests an upcoming increase in demand for certain products during a holiday season, retailers can preemptively raise prices, capitalizing on the expected surge in consumer interest.
In summary, analyzing consumer behavior and trends through AI-driven price optimization techniques enables retailers to make informed pricing decisions that can significantly enhance sales performance and improve overall profitability. By understanding how customers respond to pricing changes and leveraging predictive analytics, retailers can position themselves competitively in a rapidly evolving market.

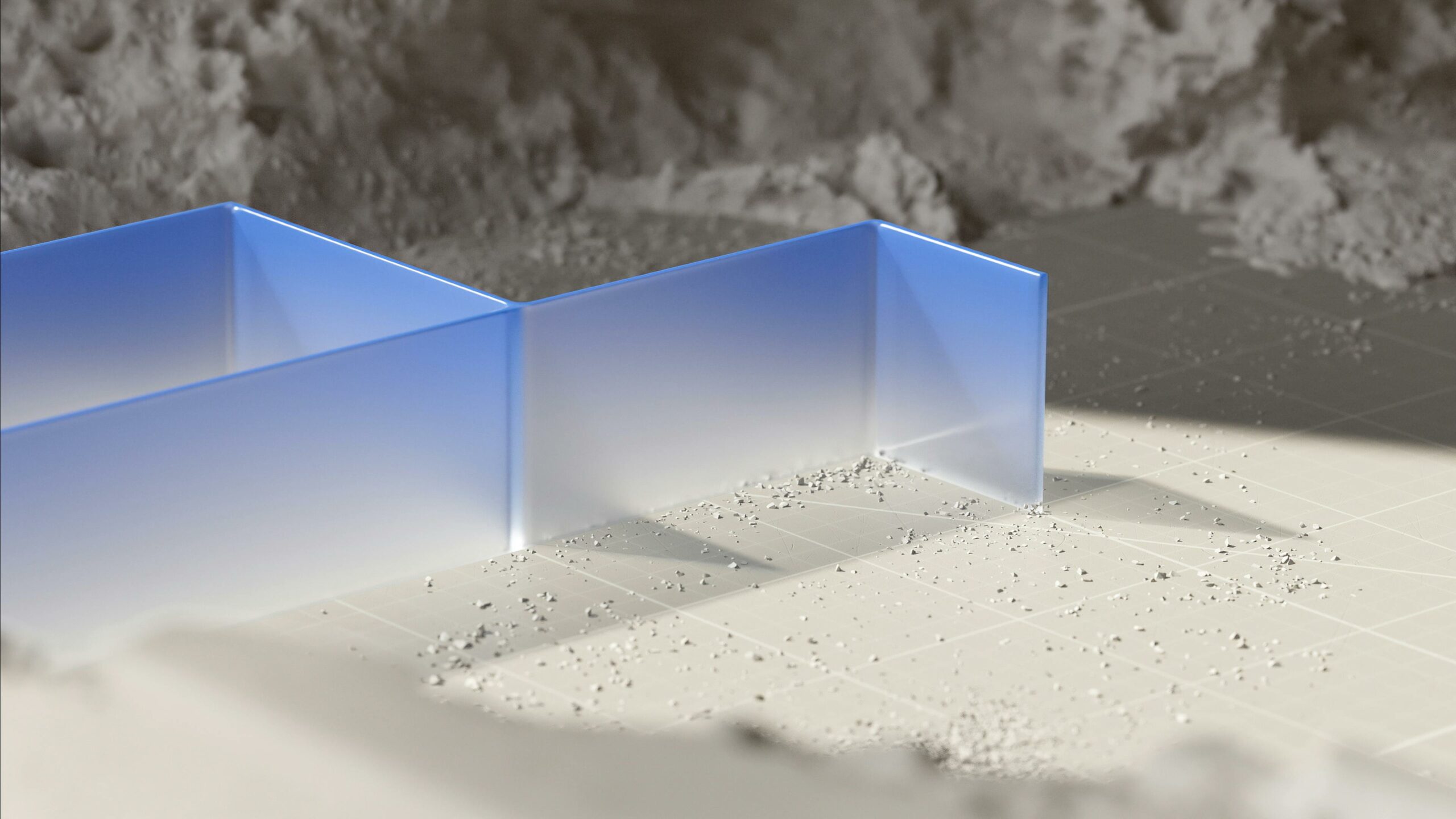
Maximizing Profit Margins
To maximize profit margins, retailers are increasingly turning to AI-driven price optimization techniques that analyze various factors influencing consumer behavior and market trends. By leveraging machine learning algorithms, these systems can process vast datasets that include historical sales data, competitor pricing, seasonal trends, and consumer purchasing patterns. This comprehensive analysis allows retailers to identify optimal pricing strategies that not only attract customers but also enhance profitability.
One of the key approaches in price optimization is the use of elasticity modeling, which helps retailers understand how changes in price affect demand. By determining the price sensitivity of different customer segments, businesses can tailor their pricing strategies to maximize revenue without alienating price-sensitive buyers. For instance, during peak shopping seasons or promotional events, retailers can strategically raise prices on high-demand items, knowing that consumers are less likely to be deterred from purchasing.
Moreover, AI systems can implement A/B testing for pricing strategies, allowing retailers to evaluate the effectiveness of different price points in real time. By comparing sales performance across various pricing scenarios, retailers can refine their strategies based on empirical evidence, ensuring that they are making data-driven decisions that align with market dynamics.
Another vital component of maximizing profit margins is understanding consumer preferences and behavior. By analyzing data from loyalty programs, social media, and online browsing habits, retailers can identify which products are trending, and adjust their pricing accordingly. For example, if a particular category of products is gaining popularity, retailers can increase prices in anticipation of higher demand, thereby enhancing their profit margins.
Additionally, integrating price optimization algorithms with inventory management systems can further optimize profitability. When stock levels are integrated into pricing strategies, retailers can adjust prices based on available inventory, reducing the chances of overstock or stockouts. This ensures that retailers not only meet customer demand but also maintain optimal pricing that reflects current market conditions.
Finally, ongoing monitoring and refinement of pricing strategies are essential. AI systems can provide real-time insights and alerts on competitor pricing changes, market shifts, and emerging trends, enabling retailers to stay agile and responsive in a fast-paced retail environment. By continuously optimizing their pricing strategies based on the latest data, retailers can ensure sustainable profit margins and maintain a competitive edge in the marketplace.
Store Operations and Management
AI-Powered Analytics for Operational Efficiency
Workforce Management
AI-powered analytics is revolutionizing workforce management in the retail sector by providing data-driven insights that enhance operational efficiency. Traditional workforce management methods often rely on historical data and manual scheduling, which can lead to inefficiencies, understaffing, or overstaffing. Conversely, AI leverages vast amounts of data to create more accurate staffing models that adapt to real-time conditions.
For instance, AI systems analyze customer traffic patterns, sales data, and external factors such as weather conditions or local events to predict peak shopping times. By employing machine learning algorithms, retailers can optimize staff schedules to ensure that the right number of employees are available when demand is highest, thereby improving customer service and minimizing labor costs. This predictive capability allows retailers to dynamically adjust workforce levels based on anticipated needs, reducing unnecessary labor expenditures and enhancing overall productivity.
Moreover, AI can facilitate employee scheduling by considering employee availability, skills, and preferences, leading to more satisfied and motivated staff. Tools that integrate AI can provide managers with dashboards that display optimal schedules, making it easier to allocate resources where they are needed most. Additionally, these systems can enable retailers to quickly adapt to unforeseen changes, such as sudden spikes in customer volume, ensuring that the store remains responsive and efficient.
Incorporating AI in workforce management also contributes to employee training and development. Retailers can analyze performance metrics to identify skill gaps and tailor training programs accordingly. By tracking individual employee performance through AI analytics, retailers can offer personalized feedback and development plans, fostering a more competent workforce.
Ultimately, the integration of AI in workforce management not only streamlines operations but also enhances employee engagement and customer satisfaction. As retailers continue to innovate in this area, the ability to harness AI for workforce management will become increasingly critical in maintaining a competitive edge in the dynamic retail landscape.
Store Layout Optimization
AI-powered analytics have increasingly become crucial for optimizing store layouts, significantly enhancing operational efficiency in the retail sector. By employing advanced algorithms and data analysis, retailers can gain insights into customer behavior, spatial dynamics, and product placement effectiveness.
One of the primary benefits of AI in store layout optimization is its ability to analyze vast amounts of customer data to determine the most effective arrangement of products within a store. This can include tracking how customers move through the space, which products attract the most attention, and where bottlenecks occur. By leveraging heat maps and customer journey analytics, retailers can identify high-traffic areas and strategically position high-margin or promotional items to maximize visibility and sales.
Moreover, AI can simulate different layout scenarios, allowing retailers to test various configurations before implementing changes. This predictive modeling helps in anticipating customer reactions to different arrangements, thus enabling data-driven decisions that enhance the overall shopping experience. For example, placing related products closer together can encourage cross-selling, while ensuring that essential items are easily accessible can improve customer satisfaction.
Additionally, AI systems can continuously learn from customer interactions, providing ongoing insights for layout adjustments. As consumer preferences evolve, retailers can adapt their store layouts in real time, ensuring that the shopping environment remains engaging and relevant. This adaptability not only improves sales but also fosters a more personalized shopping experience, ultimately leading to higher customer loyalty.
In summary, utilizing AI for store layout optimization equips retailers with powerful tools to refine their operational strategies, enhance customer interactions, and drive sales growth. By making informed, data-backed decisions regarding store design and product placement, retailers can create an environment that not only meets but anticipates shopper needs.
Fraud Detection and Loss Prevention
AI Solutions for Identifying Theft
In the realm of retail, loss prevention has traditionally relied on manual oversight and surveillance systems. However, the adoption of AI solutions has revolutionized this area, offering innovative methods for identifying theft and reducing shrinkage. AI technologies, particularly those utilizing machine learning algorithms, can analyze vast quantities of data from various sources, including point-of-sale transactions, video surveillance, and historical sales patterns, to identify suspicious behavior and anomalies that might indicate theft.
One of the primary methods through which AI detects potential theft is through advanced video analytics. These systems leverage computer vision to monitor live video feeds from security cameras. By training on datasets of known theft incidents, AI models can learn to recognize unusual behavior, such as a customer loitering for an extended period in a specific aisle or acting nervously while handling merchandise. These systems can trigger alerts for security personnel to intervene in real-time, thereby preventing potential theft before it occurs.
Moreover, AI can enhance the analysis of transaction data to identify patterns indicative of fraudulent activity. For instance, machine learning algorithms can flag transactions that deviate from the norm, such as unusually high returns or multiple high-value purchases in a short time frame, particularly when coupled with a history of suspicious behavior. By integrating transaction data with customer profiles, retailers can create a more comprehensive view of potential internal and external threats.
Additionally, the implementation of AI-driven mobile applications and smart devices can empower employees to contribute to loss prevention. Employees equipped with mobile devices can receive real-time alerts when AI systems detect suspicious activity, allowing them to act promptly. This proactive approach not only deters theft but also fosters a culture of accountability within the retail environment.
Another innovative AI solution involves utilizing RFID (Radio Frequency Identification) technology alongside AI analytics. RFID tags can be placed on merchandise, allowing for real-time tracking of inventory. If an item is removed from its designated location without being scanned at the checkout, the system can trigger an alert. This method not only helps in identifying theft but also improves inventory accuracy, contributing to overall operational efficiency.
Through these advanced AI solutions for identifying theft, retailers are better equipped to tackle loss prevention challenges. By leveraging technology, they can create safer shopping environments, protect their assets, and ultimately improve their bottom line. As AI continues to evolve, its integration into retail operations will likely become more sophisticated, further enhancing the capabilities of loss prevention strategies.
Anomaly Detection in Transactions
Anomaly detection in transactions is a critical component of fraud detection systems within the retail sector. Retailers face the constant challenge of protecting their revenue from fraudulent activities, which can range from return fraud to credit card chargebacks. By leveraging AI techniques, retailers can significantly enhance their ability to identify suspicious patterns and behaviors that may indicate fraudulent transactions.
AI-driven anomaly detection systems utilize machine learning algorithms to analyze vast amounts of transaction data in real-time. These systems can establish a baseline of normal transaction behavior by examining factors such as purchase frequency, transaction amounts, payment methods, and customer demographics. Once a baseline is established, the algorithms can effectively highlight deviations from this norm—such as unusually large purchases or atypical purchasing patterns—which could indicate potential fraud.
One of the advantages of using AI for anomaly detection is its ability to adapt continuously. As new data is fed into the system, the algorithms can learn and refine their understanding of what constitutes typical behavior for different customer segments. This dynamic learning capability helps reduce false positives, whereby legitimate transactions are incorrectly flagged as fraudulent, thus minimizing disruption to genuine customers.
Additionally, AI systems can incorporate a range of data sources beyond just transactional history. For example, they can analyze device information, geographical locations, and even social media activity to provide context to each transaction. This multi-dimensional approach allows retailers to gain a more comprehensive view of potential fraud risks and tailor their responses accordingly.
Furthermore, integrating anomaly detection with broader fraud detection frameworks can enhance overall security measures. For instance, when a transaction is flagged as anomalous, automated alerts can be sent to the fraud prevention team, allowing for immediate investigation. In more advanced setups, the system may even automatically halt suspicious transactions pending manual review.
Ultimately, the implementation of AI-powered anomaly detection not only aids in identifying and preventing fraudulent activities but also enhances the overall trustworthiness of the retail brand. By protecting against losses due to fraud, retailers can safeguard their revenues, improve customer satisfaction, and maintain a positive reputation in today’s competitive marketplace.
The Future of AI in Retail
Emerging Technologies
The Role of Machine Learning and Deep Learning
The integration of machine learning (ML) and deep learning (DL) technologies is set to reshape the retail landscape profoundly. Machine learning, a subset of AI, includes algorithms that can learn from and make predictions based on data. In retail, this means that businesses can analyze vast amounts of consumer data—such as shopping habits, preferences, and feedback—to improve decision-making processes and enhance customer engagement. As these algorithms become more sophisticated, retailers can derive insights that were previously unattainable, enabling them to predict trends, optimize inventory, and tailor personalized experiences to individual customers.
Deep learning, a more advanced subset of machine learning, utilizes neural networks to process complex data structures. This technology is particularly beneficial in analyzing unstructured data, such as images and text, which are increasingly relevant in the retail context. For instance, deep learning can power image recognition technologies that enable visual searches, allowing customers to upload photos of products they desire and receive recommendations for similar items available in the store. Additionally, it can enhance chatbots and virtual assistants by enabling them to understand and interpret customer inquiries more effectively, resulting in more meaningful interactions.
As retailers continue to adopt these technologies, they can expect to see significant improvements in operational efficiency and customer satisfaction. The ability to analyze customer sentiment through natural language processing (NLP), a branch of deep learning, can also guide retailers in refining their marketing strategies. By understanding how customers feel about their products and services, retailers can adjust their offerings and communications to better align with consumer expectations.
Overall, the role of machine learning and deep learning in retail is transformative, enabling companies to harness the power of data in innovative ways. As these technologies evolve, they will continue to provide retailers with powerful tools to enhance their business, improve the shopping experience, and stay competitive in a rapidly changing market.
Integration with IoT Devices
The integration of AI with Internet of Things (IoT) devices is poised to transform the retail landscape by creating an interconnected ecosystem that enhances operational efficiency and customer engagement. IoT devices, such as smart shelves, connected point-of-sale systems, and wearable technology, provide retailers with real-time data that can be analyzed and leveraged through AI algorithms. This synergy enables businesses to gain deeper insights into consumer behavior, preferences, and purchasing patterns, thereby facilitating more informed decision-making.
One of the key benefits of integrating AI with IoT in retail is the ability to automate and streamline various processes. For example, smart shelves equipped with weight sensors can monitor inventory levels in real-time. When stock levels drop below a certain threshold, the system can automatically trigger reordering processes, ensuring that products are always available without manual intervention. This not only minimizes the risk of stockouts but also optimizes inventory management, reducing excess inventory costs.
Moreover, AI-powered IoT devices can enhance the in-store experience for customers. Retailers can deploy geolocation technology through connected devices to provide personalized offers and recommendations to shoppers based on their location within the store. For instance, if a customer lingers in the electronics section, their smartphone could receive tailored promotions on related products, effectively driving sales while enriching the shopping experience.
The integration also extends to supply chain management, where IoT sensors track shipments and environmental conditions during transit. AI can analyze this data to predict potential disruptions or delays, allowing retailers to proactively address issues and optimize logistics. This results in more efficient supply chains and improved customer satisfaction due to timely deliveries.
As the technology continues to advance, the possibilities for integrating AI with IoT devices in retail are vast. Retailers must remain agile and open to adopting new technologies that enhance operational capabilities and improve customer interactions. However, it is crucial to approach this integration with a strategic mindset, ensuring that data collected from IoT devices is used responsibly and ethically, while also prioritizing customer privacy and security.
In conclusion, the convergence of AI and IoT devices presents a transformative opportunity for the retail sector, enabling enhanced operational efficiency, personalized customer experiences, and data-driven decision-making. As these technologies evolve and become more intertwined, retailers that embrace them will be better positioned to thrive in an increasingly competitive market.
Ethical Considerations and Challenges
Data Privacy Issues
As AI continues to reshape the retail landscape, data privacy issues emerge as a significant challenge that retailers must navigate. With the extensive amount of data required to fuel AI algorithms—such as customer purchase histories, preferences, and behavioral patterns—retailers are tasked with ensuring that this information is collected, stored, and processed ethically and securely.
One of the primary concerns involves the potential for unauthorized access to sensitive information. Retailers must implement robust cybersecurity measures to protect customer data from breaches, which can lead to severe reputational damage and legal repercussions. This necessitates a commitment to not only safeguarding data but also being transparent with customers about how their information is being utilized.
Moreover, compliance with regulations such as the General Data Protection Regulation (GDPR) in Europe and the California Consumer Privacy Act (CCPA) in the United States is critical. These laws impose strict guidelines on data collection, usage, and the rights of consumers, including the right to access, correct, and delete their personal information. Retailers must ensure their AI systems are designed to comply with these regulations, which may require modifications to existing data processing frameworks.
In addition to legal requirements, retailers face the challenge of building customer trust. Customers are becoming increasingly aware of their privacy rights and are more likely to support brands that prioritize data protection and ethical AI practices. Retailers must adopt a customer-centric approach, clearly communicating their data privacy policies and the measures taken to protect personal information. This not only fosters trust but can also enhance customer loyalty, leading to a more personalized shopping experience without compromising ethical standards.
Lastly, addressing biases in AI algorithms is an ethical consideration that retailers must confront. AI systems can unintentionally perpetuate existing biases present in the data they are trained on. For instance, if a retailer’s recommendation engine is trained predominantly on data from a specific demographic, it may fail to provide equitable product suggestions to a more diverse customer base. Retailers need to be vigilant in auditing their algorithms to ensure fairness, transparency, and inclusivity, which ultimately contributes to a more positive and equitable shopping environment.
Navigating these ethical considerations and challenges requires a proactive approach from retailers. Engaging in ongoing dialogues about data privacy, investing in secure technologies, and fostering an inclusive AI design process are all necessary steps to ensure that the future of AI in retail aligns with ethical practices and customer expectations. By addressing these issues, retailers can harness the power of AI while upholding the principles of trust and integrity in their customer relationships.
Ensuring Fairness and Transparency in AI Algorithms
As AI continues to permeate the retail sector, ensuring fairness and transparency in AI algorithms becomes increasingly critical. The reliance on algorithms for decision-making processes can inadvertently introduce biases that affect various aspects of retail operations, from customer service to inventory management. One of the primary ethical concerns centers around the data used to train these algorithms. If the training data is unbalanced or reflects existing societal biases, the AI systems may perpetuate or even exacerbate these inequalities, leading to unfair practices such as discriminatory pricing or biased product recommendations.
Transparency in AI algorithms is essential for building trust among consumers and stakeholders. Retailers must be able to explain how their AI systems make decisions, particularly in sensitive areas such as pricing, marketing, and customer interactions. This need for transparency is compounded by the complexity of many machine learning models, which can appear as „black boxes“ with decision-making processes that are difficult to understand. As a result, there is a growing call for clearer guidelines and standards that mandate explainability in AI models used within retail.
Implementing fairness and transparency measures involves several strategies. First, retailers can invest in diverse datasets that accurately represent their customer base, thereby reducing the risk of bias in algorithmic predictions. Regular audits of AI systems can also be conducted to assess for any discriminatory outcomes. Additionally, involving a diverse group of stakeholders in the development and deployment of AI systems can lead to more equitable outcomes. This approach ensures that the voices of various demographics are considered, potentially mitigating biases that may not be immediately evident in the data.
Furthermore, retailers can adopt ethical AI frameworks that prioritize fairness and accountability in their operations. These frameworks may include guidelines on data governance, regular bias assessments, and the establishment of an ethics review board to oversee AI initiatives. By embracing these practices, retailers not only enhance their reputations but also contribute to a more equitable retail landscape.
In conclusion, while AI presents numerous opportunities for innovation in retail, addressing ethical considerations related to fairness and transparency is paramount. As the industry navigates these challenges, proactive measures will be essential in fostering consumer trust, promoting inclusivity, and ensuring that AI serves as a force for good in the retail environment.
Conclusion
Summary of the Impact of AI on the Retail Industry
The integration of artificial intelligence (AI) into the retail industry has significantly transformed how businesses operate and engage with consumers. AI technologies enhance operational efficiency, drive customer engagement, and optimize supply chain management, resulting in a more streamlined and personalized shopping experience. Retailers leveraging AI can analyze vast amounts of data to understand customer preferences, forecast demand, and make data-driven decisions that improve inventory management and reduce costs. The use of recommendation engines, personalized marketing campaigns, and virtual assistants has revolutionized how retailers connect with their customers, fostering loyalty and increasing sales.
Moreover, AI’s capabilities extend into pricing strategies, allowing retailers to implement dynamic pricing models that respond to market conditions and consumer behaviors. This adaptability not only maximizes profit margins but also enhances competitive positioning in a rapidly changing retail landscape. Additionally, AI-powered analytics facilitate better store operations, from workforce management to layout optimization, ensuring that retailers can meet consumer demands efficiently.
The impact of AI on loss prevention through fraud detection and anomaly identification is another critical area where retailers benefit, significantly reducing shrinkage and related losses. As the retail sector continues to embrace AI technologies, the overall customer experience is set to improve, paving the way for innovative shopping solutions that anticipate and cater to consumer needs.
B. Potential Future Developments and Innovations in Retail AI
Looking ahead, the future of AI in retail is vibrant with the potential for groundbreaking innovations. As machine learning and deep learning technologies advance, they will enable even more sophisticated consumer insights and predictive analytics. The integration of AI with the Internet of Things (IoT) will facilitate real-time data collection from connected devices, providing retailers with unparalleled visibility into customer behavior and inventory levels.
However, as the reliance on AI increases, ethical considerations regarding data privacy, algorithmic fairness, and transparency must be addressed. Retailers will need to ensure that their AI systems are designed with ethical guidelines in mind, promoting responsible usage of consumer data and maintaining trust with their customers. By navigating these challenges, the retail industry can harness the full potential of AI, driving innovation while safeguarding consumer interests.
In conclusion, the ongoing evolution of AI technologies promises to reshape the retail landscape, creating new opportunities for growth and enhancing the overall shopping experience. As retailers continue to adapt to these technological advancements, the industry will likely witness further transformation, fostering a dynamic environment that prioritizes customer satisfaction and operational excellence.
Potential Future Developments and Innovations in Retail AI
As we look towards the future, the potential developments and innovations in AI within the retail sector are both exciting and transformative. Retailers are likely to continue leveraging advanced technologies to enhance operational efficiency, customer satisfaction, and overall business performance. One significant area of growth is the integration of AI with emerging technologies such as augmented reality (AR) and virtual reality (VR), which can create immersive shopping experiences. Imagine customers trying on clothes virtually or visualizing how furniture would look in their homes before making a purchase. This fusion of AI with AR/VR can significantly reduce return rates and improve customer satisfaction.
Additionally, the evolution of machine learning and deep learning algorithms will enable even more sophisticated predictive analytics. Retailers will increasingly harness these technologies to analyze vast amounts of data, gaining insights into consumer behavior and preferences that were previously unattainable. This will result in more accurate demand forecasting, allowing businesses to streamline their inventory and improve supply chain efficiency.
The Internet of Things (IoT) will also play a crucial role in the future of retail AI. As more devices become interconnected, retailers will be able to collect real-time data from various sources, enhancing their decision-making processes. For instance, smart shelves equipped with sensors can monitor stock levels in real time and automatically place orders when inventory dips below a certain threshold. This capability will further optimize inventory management and reduce operational costs.
However, as these technologies evolve, so too do the ethical considerations surrounding their use. Data privacy will remain a paramount concern for consumers and regulators alike. Retailers must navigate the complexities of data collection and usage while ensuring they protect consumer information. Moreover, as AI systems become more prevalent, maintaining fairness and transparency in AI algorithms will be critical to avoid biases that could adversely affect consumer experiences and outcomes.
In conclusion, the future of AI in retail is poised for significant advancements that will reshape the industry. From enhanced customer experiences through AR/VR to optimized operations via IoT and predictive analytics, the potential for innovation is vast. However, the path forward will require careful consideration of ethical implications, ensuring that the benefits of these technologies are realized while maintaining trust and transparency with consumers. As retailers continue to embrace AI, the landscape of retail will undoubtedly evolve, creating new opportunities and challenges in equal measure.