Introduction to AI and Cybersecurity
Artificial Intelligence (AI) has become a game-changer in the field of cybersecurity, revolutionizing how organizations defend against cyber threats. As the digital landscape grows increasingly complex, traditional cybersecurity measures are no longer sufficient to protect sensitive data and systems. This is where AI steps in, offering advanced capabilities to detect, respond to, and mitigate cyber attacks in real-time.
The marriage of AI and cybersecurity brings a new level of sophistication and efficiency to defensive strategies. By leveraging AI technologies, organizations can enhance their threat detection and response mechanisms, fortify vulnerability management processes, and deploy cutting-edge behavioral analytics to proactively combat security breaches. As cybercriminals continue to evolve and diversify their attack methods, AI serves as a powerful ally in the ongoing battle to safeguard digital assets.
In this chapter, we will explore the pivotal role that AI plays in cybersecurity, delving into its various applications such as threat detection, automated incident response, vulnerability management, and behavioral analytics. Additionally, we will examine the challenges and ethical considerations associated with AI implementation in cybersecurity, highlighting the importance of addressing issues such as bias in algorithms, privacy concerns, and the susceptibility of AI to adversarial attacks. Through case studies and a discussion of AI tools and technologies, we will showcase real-world examples of AI in action within cybersecurity frameworks, emphasizing its tangible benefits and effectiveness.
As we navigate through the intricate landscape of AI and cybersecurity, it is crucial to stay abreast of emerging trends and future developments in this dynamic field. By embracing innovative AI-driven solutions and fostering a culture of continuous innovation, organizations can build robust cybersecurity defenses that are agile, adaptive, and resilient in the face of evolving cyber threats. The convergence of AI and cybersecurity represents a transformative shift in how we approach defense strategies, underscoring the imperative for organizations to proactively incorporate AI technologies into their security protocols.
The Role of AI in Cybersecurity
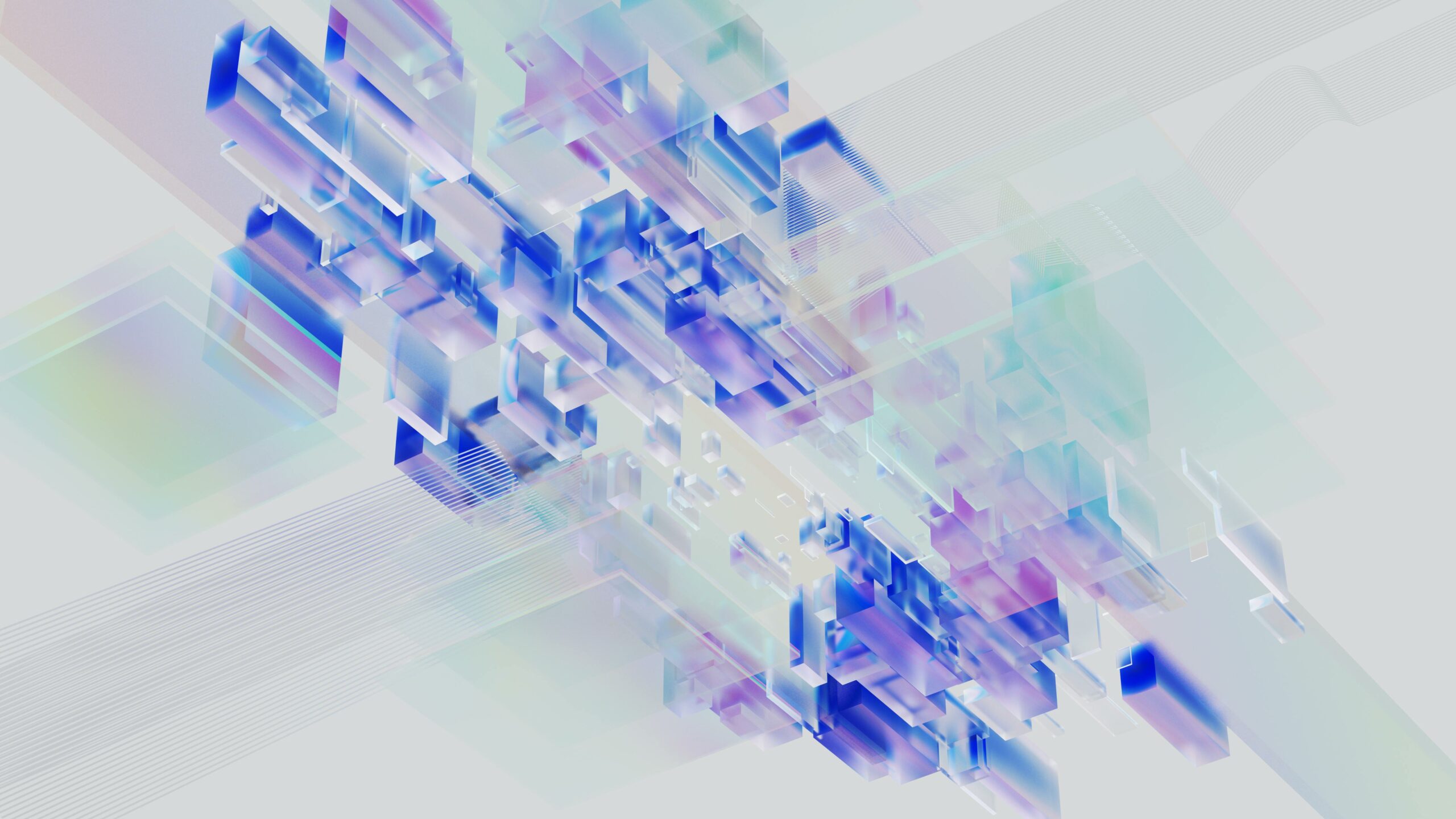
Detection and Response
AI-powered threat detection
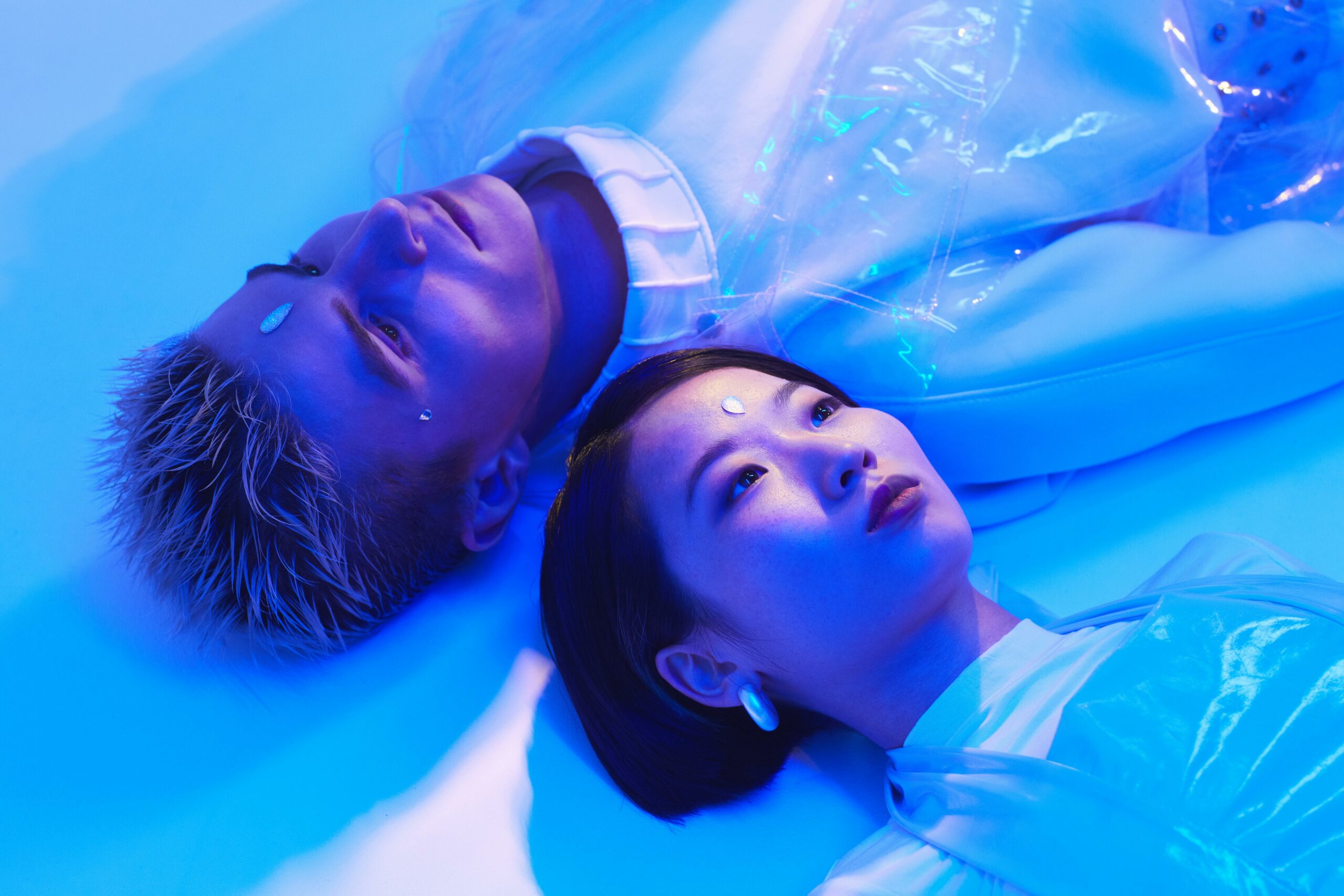
AI plays a crucial role in enhancing threat detection capabilities in cybersecurity. By leveraging AI-powered algorithms, organizations can bolster their defenses against a wide range of cyber threats. One of the key advantages of AI in threat detection is its ability to analyze vast amounts of data in real-time, which would be impossible for human analysts to process quickly and accurately.
AI-powered threat detection systems can continuously monitor network activities, user behavior, and system logs to identify potential security incidents. These systems can detect patterns and anomalies that might indicate a cyber attack or unauthorized access. By using machine learning algorithms, AI can adapt and learn from new data to improve detection accuracy over time.
Moreover, AI can automate the process of correlating different security events to provide a holistic view of potential threats. By integrating various data sources and applying advanced analytics, AI can prioritize alerts based on the level of risk they pose to the organization. This helps security teams focus on investigating and responding to the most critical threats first, reducing response times and minimizing the impact of cyber attacks.
Overall, AI-powered threat detection not only enhances the efficiency and effectiveness of cybersecurity operations but also empowers organizations to stay ahead of sophisticated cyber threats in today’s rapidly evolving threat landscape.
Automated incident response
Automated incident response is a critical aspect of utilizing AI in cybersecurity defense strategies. In the face of rapidly evolving cyber threats, the ability to respond to incidents in real-time is essential to minimize potential damage and mitigate risks effectively.
AI technologies can automate incident response processes by leveraging machine learning algorithms to analyze and interpret vast amounts of data at a speed and scale that surpasses human capabilities. Through continuous monitoring and analysis of network activities, AI systems can swiftly identify anomalous behavior patterns that may indicate a security incident.
Once a potential threat is detected, automated incident response mechanisms can be triggered to execute predefined response actions, such as isolating affected systems, blocking malicious traffic, or initiating remediation processes. By streamlining response actions and reducing the time to contain threats, AI-driven automation plays a crucial role in enhancing the overall efficiency and effectiveness of cybersecurity operations.
Furthermore, automated incident response not only accelerates the detection and containment of security incidents but also enables security teams to focus their efforts on more complex and strategic tasks that require human intervention. By automating repetitive and time-consuming response activities, organizations can improve their incident response capabilities and better safeguard their digital assets against a wide range of cyber threats.
Vulnerability Management
AI in identifying and prioritizing vulnerabilities
AI plays a crucial role in identifying and prioritizing vulnerabilities in cybersecurity. Traditional methods of vulnerability management often struggle to keep pace with the rapidly evolving threat landscape. AI offers a more proactive and efficient approach to identifying vulnerabilities before they can be exploited by malicious actors.
One key advantage of using AI in vulnerability management is the ability to process and analyze vast amounts of data at a speed and scale that would be impossible for humans alone. AI algorithms can quickly scan through code, network traffic, and system logs to pinpoint potential weaknesses in an organization’s security posture.
Moreover, AI can help in prioritizing vulnerabilities based on their severity and potential impact on the organization. By assigning risk scores to each vulnerability, AI systems can assist cybersecurity teams in focusing their efforts on addressing the most critical issues first. This not only saves time and resources but also ensures that the most pressing security gaps are addressed promptly.
Additionally, AI-powered vulnerability management systems can provide recommendations for remediation strategies, taking into account factors such as the organization’s infrastructure, security policies, and industry regulations. This proactive guidance can help organizations streamline their patch management processes and improve overall cybersecurity resilience.
In conclusion, AI’s role in identifying and prioritizing vulnerabilities is essential for enhancing cybersecurity defense mechanisms. By leveraging AI capabilities in vulnerability management, organizations can stay one step ahead of cyber threats and better protect their sensitive data and assets from potential attacks.
Patch management with AI
Patch management is a critical aspect of cybersecurity, ensuring that software vulnerabilities are promptly addressed to mitigate the risk of cyber attacks. AI is revolutionizing patch management by offering more efficient and effective ways to identify and prioritize vulnerabilities for timely patching.
One of the key advantages of using AI in patch management is its ability to analyze vast amounts of data quickly and accurately. Machine learning algorithms can scan software systems to identify vulnerabilities and assess their severity based on factors such as potential impact and exploitability. This automated process enables organizations to stay ahead of emerging threats and prioritize patches based on the level of risk they pose to the system.
Moreover, AI can help streamline the patch management process by automating certain tasks, such as patch deployment and verification. By leveraging AI-powered tools, organizations can reduce the time and resources required to apply patches across their systems, minimizing the window of opportunity for attackers to exploit known vulnerabilities.
Another benefit of using AI in patch management is its predictive capabilities. By analyzing historical data and trends, AI algorithms can anticipate potential vulnerabilities that may arise in the future, allowing organizations to proactively address them before they can be exploited by cybercriminals.
Overall, AI is transforming patch management by making the process more efficient, accurate, and proactive. By harnessing the power of AI technologies, organizations can strengthen their cybersecurity defenses and protect their systems against evolving cyber threats.
Behavioral Analytics
AI for user behavior analysis
Behavioral analytics is a crucial aspect of cybersecurity, as it focuses on understanding and predicting the behavior of users within a network environment. By leveraging AI, organizations can gain valuable insights into normal behavioral patterns, detect anomalies, and identify potential security threats in real-time.
AI plays a significant role in user behavior analysis by continuously monitoring and analyzing vast amounts of data to establish a baseline of normal user behavior. Through machine learning algorithms, AI can identify deviations from established patterns, such as unusual login times, access to unauthorized resources, or irregular data transfer activities. These anomalies are flagged as potential security incidents, enabling security teams to investigate and respond promptly before any damage occurs.
Moreover, AI-powered user behavior analysis can help organizations in detecting insider threats, where employees or authorized users misuse their access privileges to compromise sensitive data or systems. By establishing user behavior profiles and applying AI algorithms to detect deviations from these profiles, organizations can proactively identify and mitigate insider threats before they escalate into full-blown security breaches.
In addition to anomaly detection, AI can assist in predicting potential insider threats by analyzing historical user behavior data and identifying trends or patterns that may indicate malicious intent. By employing predictive analytics, organizations can take preemptive measures to prevent security incidents before they occur, thereby enhancing their overall cybersecurity posture.
Overall, AI-driven user behavior analysis is a valuable tool in modern cybersecurity operations, providing organizations with the ability to detect, investigate, and mitigate potential threats proactively. By leveraging AI technologies in this domain, organizations can strengthen their defenses against a wide range of cyber threats, ultimately safeguarding their critical assets and sensitive information.
Anomaly detection using AI
Anomaly detection plays a crucial role in enhancing cybersecurity measures by identifying deviations from normal behavior within a system. AI technologies have significantly revolutionized anomaly detection, enabling organizations to proactively detect and respond to potential security threats.
AI algorithms can analyze vast amounts of data in real-time to establish patterns of normal behavior for users, devices, and networks. By leveraging machine learning and deep learning techniques, AI-powered anomaly detection systems can continuously learn and adapt to new patterns and behaviors, thereby improving detection accuracy and reducing false positives.
One of the key advantages of using AI for anomaly detection is the ability to detect subtle and sophisticated threats that may go unnoticed by traditional rule-based systems. By monitoring and analyzing a wide range of parameters and behaviors, AI can identify anomalies that indicate potential security breaches or malicious activities.
Furthermore, AI-powered anomaly detection systems can help organizations in understanding the context of anomalies and prioritizing alerts based on the level of risk they pose. This enables security teams to focus their efforts on investigating and mitigating the most critical threats promptly.
As cyber threats continue to evolve and become more sophisticated, anomaly detection using AI will play a vital role in strengthening the overall cybersecurity posture of organizations. By proactively identifying and responding to anomalies, businesses can better protect their sensitive data, systems, and networks from potential security breaches and cyber attacks.
Challenges and Ethical Considerations
Bias in AI algorithms
Bias in AI algorithms is a significant challenge in the realm of cybersecurity. AI systems are only as good as the data they are trained on, and if this data is biased in any way, it can lead to discriminatory outcomes in cybersecurity processes. For instance, if the training data for an AI threat detection system is skewed towards certain types of threats or attackers, it may result in the system being less effective at identifying other types of threats.
Moreover, bias can also seep into AI algorithms through the design choices made by developers. Preconceived notions or unconscious biases held by developers can inadvertently influence the way AI systems operate, leading to skewed results or unfair treatment of certain groups or individuals.
Addressing bias in AI algorithms requires a multi-faceted approach. It involves ensuring that the training data used is diverse, representative, and free from biases. Additionally, developers and data scientists must actively work to identify and mitigate biases in the algorithms themselves, through techniques such as bias testing, fairness-aware machine learning, and ongoing monitoring of AI systems in production.
Ethical considerations also come into play when discussing bias in AI algorithms. Ensuring fairness, transparency, and accountability in the development and deployment of AI systems is crucial to maintaining trust in these technologies, especially in sensitive domains like cybersecurity. Organizations must be vigilant in monitoring and addressing bias in their AI systems to uphold ethical standards and prevent potential harm to individuals or groups.
Privacy concerns
Privacy concerns have become a significant issue in the intersection of AI and cybersecurity. As AI technologies are increasingly utilized in cybersecurity practices, concerns about the privacy of individuals‘ data have been raised. One of the primary worries is the potential misuse or mishandling of sensitive information gathered by AI systems for cybersecurity purposes.
AI-powered cybersecurity tools often require access to large amounts of data, including personal and confidential information, to effectively detect threats and vulnerabilities. This data may include user behavior patterns, network activity, and communication content. The collection and analysis of such data raise privacy concerns, especially regarding how this information is stored, processed, and protected from unauthorized access.
Another aspect of privacy concerns in AI and cybersecurity is the risk of data breaches or leaks. If AI-powered security systems are compromised by cyber attackers, the sensitive data they handle could be exposed, leading to serious privacy violations. Additionally, there is a concern about the potential for AI algorithms to make incorrect decisions or interpretations that could impact individuals‘ privacy rights.
Furthermore, the use of AI in cybersecurity may also raise questions about transparency and accountability. The complexity of AI algorithms and the automated nature of AI systems can make it challenging to understand how decisions are made or actions are taken, leading to potential privacy risks if the processes are not transparent or accountable.
Addressing privacy concerns in the context of AI and cybersecurity requires a careful balance between leveraging the benefits of AI technologies for enhancing security measures while safeguarding individuals‘ privacy rights. Organizations and cybersecurity professionals must implement robust data protection measures, secure data handling practices, and transparent processes to mitigate privacy risks associated with AI-powered cybersecurity solutions. Additionally, regulatory compliance with data protection laws and standards is essential to ensure that privacy concerns are adequately addressed in the development and deployment of AI technologies for cybersecurity purposes.
Adversarial attacks on AI
Adversarial attacks on AI present a significant challenge in the realm of cybersecurity. These attacks target the vulnerabilities in AI systems, exploiting them to manipulate the behavior of the algorithms. Adversarial attacks can have serious consequences, leading to misclassification of data, evasion of detection mechanisms, or even causing AI systems to make incorrect decisions.
One of the key issues with adversarial attacks is the ability to deceive AI models without being detected. Attackers can introduce subtle, carefully crafted perturbations to input data, causing the AI system to misinterpret the information. For example, in image recognition systems, adding imperceptible noise to an image can trick the AI into misclassifying objects.
Moreover, adversarial attacks can undermine the trustworthiness and reliability of AI systems. If attackers can consistently manipulate AI algorithms, it can erode confidence in the technology, hindering its widespread adoption in critical applications such as cybersecurity.
Addressing adversarial attacks requires a multi-faceted approach. Incorporating robust security measures into the design and implementation of AI systems is essential to mitigate vulnerabilities. Techniques such as adversarial training, which involves exposing AI models to adversarial examples during the training phase, can help enhance the resilience of the algorithms against such attacks.
Furthermore, ongoing research is vital to stay ahead of evolving adversarial techniques. By continually analyzing and understanding the tactics used by attackers, the cybersecurity community can develop proactive defense strategies to bolster the security of AI systems.
In conclusion, while adversarial attacks pose a significant challenge to AI in cybersecurity, proactive measures and collaborative efforts can strengthen the resilience of AI systems against such threats. By addressing the vulnerabilities and enhancing the security posture of AI algorithms, organizations can better defend against adversarial attacks and ensure the integrity of their cybersecurity defenses.
AI Tools and Technologies in Cybersecurity
Machine Learning for Malware Detection
Machine learning has emerged as a powerful tool in the field of cybersecurity, particularly in the realm of malware detection. By leveraging algorithms and statistical models, machine learning enables systems to learn from and make decisions based on data without being explicitly programmed. This capability has significantly enhanced the ability to detect and combat malware threats in various ways.
One key application of machine learning in malware detection is in the realm of anomaly detection. Traditional signature-based anti-malware tools are limited in their effectiveness against new and unknown threats. Machine learning models, on the other hand, can analyze patterns in data and identify anomalies that may indicate the presence of malware. By training on large datasets of both benign and malicious samples, machine learning algorithms can learn to recognize patterns of behavior associated with different types of malware.
Another important use of machine learning in malware detection is in the realm of file analysis. Machine learning models can be trained to analyze file attributes and behavior to determine the likelihood of a file being malicious. By examining features such as file size, file type, and code structure, machine learning algorithms can make informed decisions about the potential threat posed by a particular file.
Furthermore, machine learning can also be employed in the analysis of network traffic and communication patterns to detect signs of malicious activity. By monitoring network behavior and identifying deviations from normal patterns, machine learning algorithms can flag suspicious activities that may indicate the presence of malware or a cyber attack.
Overall, the application of machine learning in malware detection represents a significant advancement in cybersecurity defense strategies. By harnessing the power of algorithms and data analysis, organizations can bolster their security posture and better protect against the constantly evolving landscape of cyber threats.
Natural Language Processing for Threat Intelligence
Natural Language Processing (NLP) plays a crucial role in enhancing threat intelligence within cybersecurity frameworks. By leveraging NLP techniques, organizations can streamline the process of extracting valuable insights from unstructured textual data sources such as security reports, social media feeds, and threat intelligence feeds.
One of the primary applications of NLP in threat intelligence is the automation of information gathering and analysis. NLP algorithms can be trained to sift through vast amounts of data to identify relevant threat indicators, such as malware signatures, suspicious IP addresses, or phishing URLs. This automation not only saves time and resources but also enables security analysts to focus on more strategic tasks, such as threat mitigation and incident response.
Furthermore, NLP can facilitate the categorization and contextualization of threat data, enabling organizations to better understand the nature and severity of potential risks. By extracting key entities, relationships, and sentiments from textual data, NLP models can provide valuable context that enhances the accuracy and relevance of threat intelligence reports.
Another significant benefit of using NLP in threat intelligence is its ability to support multilingual analysis. As cyber threats transcend geographical boundaries, organizations need to monitor and analyze information in multiple languages to stay ahead of evolving risks. NLP tools can be customized to process and interpret text in various languages, enabling a more comprehensive and global approach to threat intelligence.
In conclusion, the integration of Natural Language Processing technologies in cybersecurity operations empowers organizations to improve the speed, accuracy, and efficiency of their threat intelligence processes. By automating data collection, analysis, and interpretation tasks, NLP contributes to a more proactive and effective cybersecurity posture, enabling organizations to better anticipate, detect, and respond to cyber threats in real-time.
Predictive Analytics for Risk Assessment
Predictive analytics is a powerful tool in the realm of cybersecurity, leveraging AI technologies to enhance risk assessment processes. By utilizing historical data, statistical algorithms, and machine learning techniques, predictive analytics can forecast potential future cybersecurity threats and vulnerabilities.
One of the key benefits of predictive analytics in risk assessment is its ability to identify patterns and trends that might indicate a security breach before it occurs. By analyzing vast amounts of data, predictive analytics can uncover anomalies or deviations from normal patterns, allowing cybersecurity professionals to take proactive measures to mitigate risks.
Moreover, predictive analytics can help in prioritizing security efforts by focusing on the most critical vulnerabilities or threats based on the likelihood of occurrence and potential impact. This enables organizations to allocate their resources more effectively and efficiently, thereby strengthening their overall cybersecurity posture.
In essence, predictive analytics serves as a valuable tool for organizations looking to stay ahead of cyber threats and anticipate security challenges before they materialize. By harnessing the power of AI and data analytics, predictive analytics can significantly enhance risk assessment processes and bolster cybersecurity defenses in an increasingly complex and dynamic threat landscape.
Case Studies: AI Implementation in Cybersecurity
Use of AI in network security
Network security is a critical aspect of cybersecurity, and the integration of AI technologies has greatly enhanced the ability to detect and respond to threats in real-time. One of the significant use cases of AI in network security is the deployment of AI-powered network intrusion detection systems (NIDS). These systems continuously monitor network traffic, analyze patterns, and identify any malicious activities or anomalies that could indicate a potential cyber attack.
AI algorithms can quickly process vast amounts of network data, enabling them to detect sophisticated threats that traditional security measures might overlook. Machine learning algorithms, in particular, can learn and adapt to new attack vectors, making them highly effective in detecting zero-day attacks and other advanced threats.
Moreover, AI-driven NIDS can also automate incident response processes, such as isolating compromised systems, blocking suspicious traffic, and alerting security teams in real-time. This rapid response capability is crucial in minimizing the impact of cyber attacks and preventing them from spreading across the network.
Another key benefit of using AI in network security is its ability to conduct predictive analysis based on historical data and ongoing network activities. By identifying patterns of behavior and potential vulnerabilities, AI systems can proactively recommend security measures to mitigate risks and strengthen the overall security posture of an organization’s network infrastructure.
Overall, the implementation of AI in network security not only enhances threat detection and incident response capabilities but also empowers organizations to stay ahead of cyber threats in today’s dynamic and evolving threat landscape.
AI applications in endpoint security
AI applications in endpoint security have revolutionized the way organizations protect their devices and data. By leveraging AI technologies, companies can enhance threat detection, response capabilities, and overall security posture at the endpoint level.
One of the key areas where AI is making significant strides in endpoint security is in the realm of threat detection. Traditional signature-based antivirus solutions are no longer sufficient to combat the rapidly evolving threat landscape. AI-powered endpoint security solutions utilize machine learning algorithms to detect and respond to both known and unknown threats in real-time. These solutions can identify patterns and anomalies indicative of malicious activity, enabling proactive threat mitigation before significant damage occurs.
Moreover, AI plays a crucial role in endpoint security by enabling behavioral analytics. By continuously monitoring user behavior and endpoint activities, AI algorithms can establish baseline behavior profiles for individual users and devices. Any deviations from these baselines can raise red flags for potential security incidents, allowing security teams to investigate and respond promptly.
Furthermore, AI enhances endpoint security through automated incident response capabilities. In the event of a security breach or suspicious activity, AI algorithms can trigger automated response actions to contain the threat, isolate affected endpoints, and prevent lateral movement within the network. This rapid response mechanism is critical in minimizing the impact of security incidents and reducing dwell time.
Overall, the integration of AI in endpoint security not only strengthens threat detection and response capabilities but also enables organizations to proactively defend against sophisticated cyber threats. As cyber adversaries continue to evolve their tactics, leveraging AI technologies in endpoint security will be essential to ensuring comprehensive protection for endpoints and critical data assets.
Future Trends in AI and Cybersecurity
Advancements in AI for threat hunting
One of the future trends in AI and cybersecurity is the advancements in AI for threat hunting. Threat hunting involves proactively searching for security threats within an organization’s network and endpoints. Traditionally, threat hunting has been a manual and time-consuming process for cybersecurity analysts. However, with the advancements in AI technologies, threat hunting is becoming more efficient and effective.
AI is increasingly being used to augment human capabilities in threat hunting by analyzing vast amounts of data in real-time to identify potential security threats. Machine learning algorithms can automatically detect patterns and anomalies that may indicate malicious activity within the network. This allows cybersecurity teams to stay ahead of emerging threats and respond more quickly to incidents.
Moreover, AI can also help in prioritizing threats based on their severity and likelihood of exploitation. By leveraging predictive analytics, AI can assess the risk associated with different threats and assist security analysts in focusing their efforts on addressing the most critical issues first.
As AI continues to evolve, we can expect to see more sophisticated applications of AI in threat hunting. For example, the integration of AI with threat intelligence feeds can provide security teams with real-time insights into the latest cyber threats and trends, enabling proactive threat hunting strategies.
Overall, the advancements in AI for threat hunting signal a shift towards more proactive and predictive cybersecurity approaches. By harnessing the power of AI technologies, organizations can strengthen their cybersecurity posture and better protect against evolving cyber threats.
Rise of AI-powered security operations centers
As the realm of cybersecurity continues to evolve, there is a notable rise in the integration of AI technologies within security operations centers (SOCs) worldwide. These AI-powered security operations centers are revolutionizing the way organizations detect, respond to, and mitigate cyber threats in real-time.
One key aspect driving the rise of AI-powered SOCs is the ability of AI algorithms to analyze vast amounts of data swiftly and accurately. By leveraging machine learning and deep learning algorithms, these SOCs can process and interpret security data at a speed and scale unattainable by human analysts alone. This enables organizations to detect and respond to threats proactively, reducing the time taken to identify and remediate security incidents.
Another significant advantage of AI-powered SOCs is their capability to enhance threat intelligence. By continuously analyzing and correlating data from various sources, including network traffic, endpoints, and cloud environments, these SOCs can identify patterns and trends indicative of potential security breaches. This proactive approach empowers organizations to stay ahead of cyber adversaries and preemptively protect their digital assets.
Furthermore, AI-powered SOCs are instrumental in automating routine security tasks and processes, allowing security teams to focus on more strategic initiatives. By automating threat detection, incident response, and even decision-making processes, these SOCs streamline security operations and improve overall efficiency. Additionally, AI technologies can learn from past incidents and adapt their responses to emerging threats, making them invaluable assets in the fight against sophisticated cyber attacks.
Looking ahead, the rise of AI-powered security operations centers is set to continue, with advancements in AI algorithms, cloud computing, and data analytics driving further innovation in cybersecurity defense. As organizations confront increasingly complex and relentless cyber threats, embracing AI technologies within their SOCs will be essential to strengthening their security posture and safeguarding their digital infrastructure.
Conclusion
Recap of the significance of AI in enhancing cybersecurity
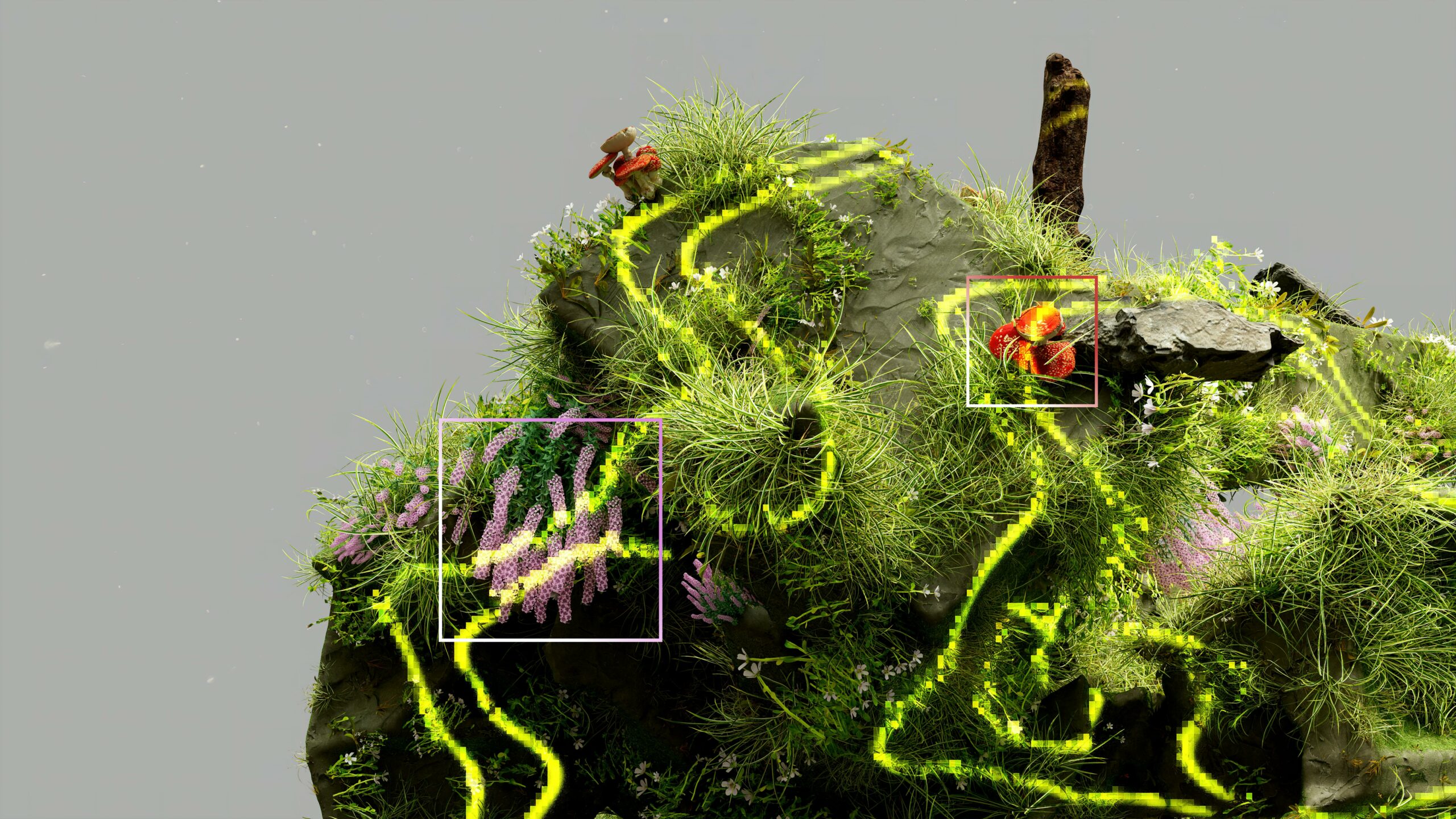
In conclusion, the significance of AI in enhancing cybersecurity cannot be overstated. AI technologies have revolutionized the way threats are detected, responses are automated, vulnerabilities are managed, and risks are assessed in the realm of cybersecurity. The ability of AI to analyze vast amounts of data at speeds far exceeding human capacity has provided organizations with a powerful tool to combat increasingly sophisticated cyber threats.
By leveraging AI for threat detection, incident response, vulnerability management, and behavioral analytics, cybersecurity professionals can stay ahead of malicious actors and protect sensitive data and systems effectively. The advancements in machine learning, natural language processing, and predictive analytics have further strengthened the cybersecurity posture of organizations, enabling them to proactively defend against evolving threats.
As we navigate the complex cybersecurity landscape, it is essential for organizations to embrace AI technologies and continuously innovate to adapt to emerging challenges. The call to action is clear – by integrating AI tools and technologies into cybersecurity strategies, organizations can establish robust defenses and safeguard their digital assets against a wide range of cyber threats. The future of cybersecurity lies in harnessing the power of AI to enhance threat hunting capabilities and establish AI-powered security operations centers that can effectively mitigate risks in real-time.
In this rapidly evolving digital age, where cyber threats continue to grow in complexity and scale, the adoption of AI in cybersecurity is not just a choice but a necessity. Organizations must prioritize investing in AI-driven cybersecurity solutions to stay resilient and secure in the face of persistent cyber risks. By embracing AI technologies and fostering a culture of innovation, organizations can build a strong foundation for robust cybersecurity defenses that can adapt to the ever-changing threat landscape.
The need for continuous innovation and adaptation in the face of evolving cyber threats
In the ever-evolving landscape of cybersecurity, the need for continuous innovation and adaptation is paramount. As cyber threats become more sophisticated and pervasive, organizations must stay ahead of the curve by leveraging cutting-edge technologies like artificial intelligence (AI) to bolster their defenses.
AI offers a proactive approach to cybersecurity, enabling organizations to detect and respond to threats in real-time, identify vulnerabilities before they are exploited, and analyze user behavior for early warning signs of potential breaches. However, as cybercriminals also harness the power of AI to launch attacks, the defense mechanisms must be constantly refined and updated to stay effective.
To effectively combat the dynamic nature of cyber threats, organizations must prioritize ongoing research and development in AI technologies. This includes investing in AI-driven solutions for threat detection, incident response, and risk assessment. By staying abreast of the latest advancements in AI for cybersecurity, organizations can better protect their digital assets and sensitive information from malicious actors.
In conclusion, the need for continuous innovation and adaptation in cybersecurity cannot be overstated. As technologies evolve and threat landscapes shift, organizations must embrace AI tools and technologies to fortify their defenses and stay one step ahead of cyber adversaries. By fostering a culture of innovation and proactive defense strategies, organizations can better safeguard their digital infrastructure and preserve the integrity of their operations in an increasingly interconnected world.
Call to action for organizations to embrace AI technologies for robust cybersecurity defenses
As the landscape of cyber threats continues to evolve and become more sophisticated, it is imperative for organizations to stay ahead of the curve by leveraging advanced technologies such as Artificial Intelligence (AI) to bolster their cybersecurity defenses. The call to action for organizations is clear: embrace AI technologies to enhance the resilience of their cybersecurity infrastructure.
By integrating AI-driven solutions into their cybersecurity strategies, organizations can benefit from real-time threat detection, proactive incident response, and automated vulnerability management. These capabilities not only enable faster and more accurate threat mitigation but also free up valuable resources within the organization that can be allocated to other critical tasks.
Furthermore, the use of AI in cybersecurity enables organizations to harness the power of predictive analytics, machine learning, and natural language processing to identify and respond to threats more effectively. These technologies provide a level of intelligence and automation that is essential in combating the dynamic and persistent nature of cyber threats.
In conclusion, the adoption of AI technologies in cybersecurity is not just a recommendation but a necessity in today’s digital landscape. Organizations that fail to embrace AI-driven solutions may find themselves at a significant disadvantage in defending against the ever-evolving threat landscape. It is crucial for organizations to prioritize the implementation of AI technologies to build robust cybersecurity defenses that can withstand the challenges of tomorrow.